In the bustling world of machine learning, the race for the most powerful, most insightful algorithms is always on. In this pursuit, the data annotators play the most crucial work behind the curtains. Their meticulous labeling and classification form the very foundation of ML models.
However, this essential role comes with its own side of difficulties, which could cause harm to the base of the machine learning models. In case of poor data quality, the most sophisticated algorithm could suffer and collapse.
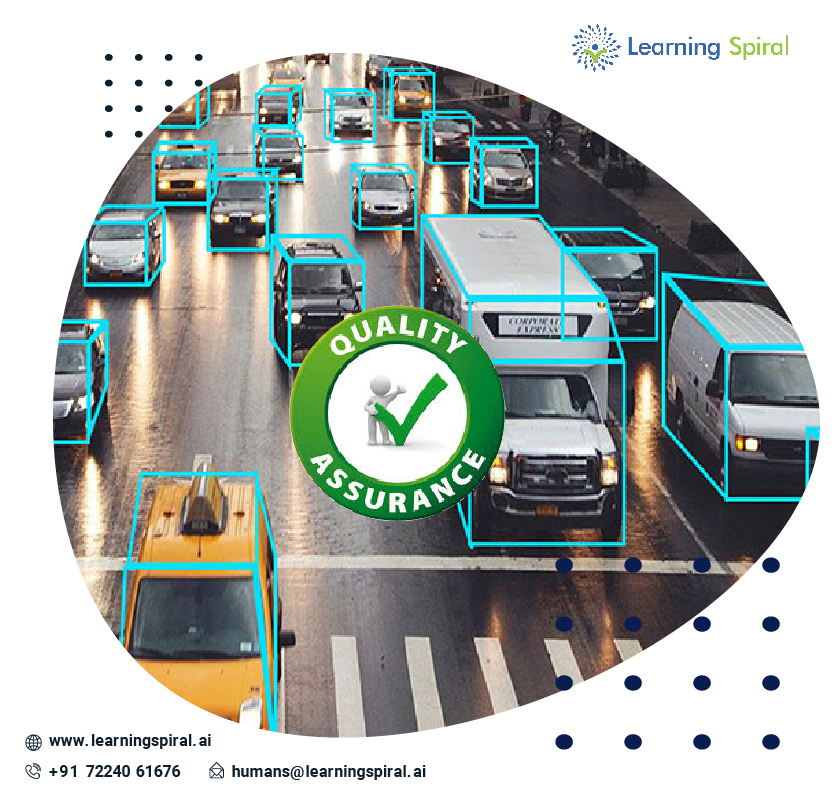
That’s where quality assurance in data annotation steps in. By creating a strong and structured procedure for quality assurance, a company can ensure the best outcomes in machine learning models.
Here are some best practices for robust quality assurance in data annotation:
1. Clear, Comprehensive Guidelines:
Before a single label is applied, the blueprint for quality must be laid out. This means establishing clear and concise annotation guidelines that meticulously define every label, address edge cases, and provide unambiguous examples. These guidelines would ensure consistent and accurate labeling throughout the project.
2. Assemble a Stellar Annotation Team:
Recruiting knowledgeable individuals with relevant domain experience and rigorous training in annotation best practices is essential. Additionally, fostering a collaborative environment where knowledge sharing and feedback are encouraged further elevates the overall quality of the work.
3. Implement Rigorous Quality Control Measures:
Robust quality assurance strategies include random sampling and double-checking of annotations, employing subject matter experts to review complex cases, and utilizing automated tools to identify inconsistencies and errors. These checks and balances catch and correct flaws before they permeate the data and ultimately harm the model’s performance.
4. Leverage Technology for Efficiency and Insights:
Quality assurance can leverage platforms equipped with automated workflows and active learning algorithms that prioritize critical data points. In the same way, real-time feedback mechanisms should be installed to streamline the process and glean valuable insights into annotation quality.
These technological aids not only save time and resources but also provide objective data to guide further improvements.
5. Embrace Continuous Improvement:
Regularly analyzing metrics like inter-annotator agreement, task completion times, and error rates provides valuable feedback that can be used to refine guidelines, enhance training, and optimize overall workflow. This iterative approach ensures that quality assurance practices evolve alongside the needs of the project, constantly striving for higher quality and greater efficiency.
By prioritizing clear guidelines, skilled teams, rigorous checks, and continuous improvement, we can confidently build the pillars of superior ML models, shaping a future where technology serves us all with both brilliance and integrity. Remember, the quality of your data determines the quality of your models.