Transfer learning is a machine learning data annotation technique where an AI model trained on one task is adapted for a somewhat related task. In simple words, instead of starting from scratch, using a pre-trained model as a starting point and fine-tuning it as per the specific task is transfer learning.
This concept has been utilized by several organizations and is increasing efficiency for data annotation requirements due to these factors:
Faster Annotation:
In a traditional data annotation process, annotators label data from scratch, which can be time-consuming. With transfer learning, a pre-trained model can be fine-tuned on a specific dataset, saving annotators time and effort.
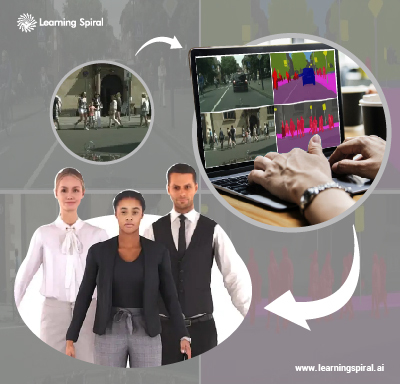
Improved Accuracy:
Pre-trained models often learn useful features from a variety of data. By using these features as a foundation, you can improve the accuracy of annotations. Transfer learning helps reduce human error by providing initial predictions or suggestions for annotators to refine.
Reduced Annotator Workload:
With transfer learning, annotators don’t need to annotate every instance from the base. They can review and correct predictions made by the pre-trained model, significantly reducing the overall workload. This is particularly useful when dealing with large datasets.
Best Practices for Leveraging Transfer Learning in Data Annotation:
1. Select the Right Model:
Choose a pre-trained model that is suitable for your annotation task. The model’s architecture and the data it was trained on should be relevant to your specific dataset.
2. Fine-Tuning:
Fine-tuning is the process of adapting the pre-trained model to your dataset. This involves training the model on your labeled data while retaining the knowledge it gained from the original task. Carefully design the fine-tuning process to achieve the desired results.
3. Quality Assurance:
Implement a quality control mechanism to ensure that the annotations provided by the fine-tuned model meet the required quality standards.
4. Iterative Process:
Transfer learning for data annotation is often an iterative process. Annotators may need to fine-tune and retrain the model multiple times to achieve the desired level of accuracy and efficiency.
5. Human-in-the-Loop:
Maintain a human-in-the-loop approach to ensure the final annotations meet the desired quality. Annotators should review and validate the model’s predictions.
6. Feedback Loop:
Establish a feedback loop between annotators and the model. Annotators can provide feedback on the model’s performance, which can be used to fine-tune and improve the model further.
7. Data Augmentation:
Use data augmentation techniques to increase the diversity of your dataset and improve the model’s ability to handle a wide range of cases.
8. Regular Model Updates:
As new annotated data becomes available, use it to retrain and update the pre-trained model to keep it aligned with the evolving annotation requirements.
In conclusion, transfer learning is a powerful technique for enhancing the efficiency of data annotation. By leveraging pre-trained models and fine-tuning them for specific tasks, organizations can reduce annotation time, improve accuracy, and make the most of existing resources.