In today’s data-driven world, understanding human emotions through text is a new norm now. But, grasping emotional ordeals with the means of words on a digital screen can be crucial. This is where sentiment analysis comes into play.
By leveraging the power of Natural Language Processing (NLP), sentiment analysis allows one to discern the underlying sentiment behind textual data, such as reviews, social media posts, or customer surveys. At the heart of this technology lies a vital first step: data annotation.
Connection Between Data Annotation and Sentiment Analysis
Data annotation for sentiment analysis involves manually labeling data in such a manner that emotional information also gets relayed. This process involves human annotators meticulously labeling text snippets with their perceived sentiment, typically categorized as positive, negative, or neutral.
However, sentiment analysis delves deeper, often aiming to capture a wider spectrum of emotions, including joy, sadness, anger, and fear.
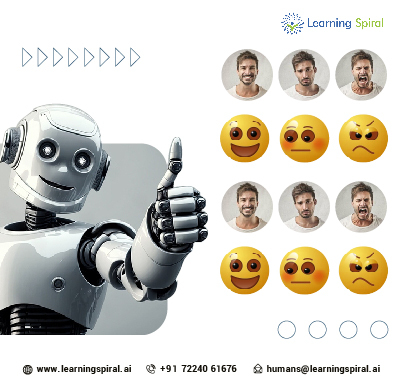
This manual labeling process is crucial for training and refining sentiment analysis models. By providing a ground truth for the model to learn from, annotated data sets the foundation for the model’s ability to interpret future, unseen data.
Challenges of Data Annotation for Sentiment Analysis:
While seemingly straightforward, data annotation for sentiment analysis presents several challenges:
- Subjectivity: Human perception of emotions is inherently subjective. The same text might evoke different emotional responses from different individuals. This necessitates ensuring a diverse pool of annotators with clear and consistent guidelines to minimize discrepancies.
- Sarcasm and Context: Language often employs sarcasm and irony, which can easily be misinterpreted by machines. Annotators need to be trained to identify and handle these nuances, considering the broader context surrounding the text, including tone, colloquialisms, and cultural references.
- Complexity of Emotions: Human emotions are multifaceted and can exist in varying intensities and combinations. Capturing the subtle differences between, say, frustration and anger requires careful consideration and well-defined annotation schemes.
Strategies for Effective Data Annotation:
To overcome the above-mentioned challenges, several strategies can be employed:
- Standardized Annotation Guidelines: Developing clear and comprehensive guidelines that define specific criteria for identifying different sentiments and emotions is essential. These guidelines should include examples and address potential ambiguities like sarcasm.
- Annotator Training: Investing in annotator training ensures consistency and reduces subjectivity. This training should cover the annotation guidelines, best practices for interpreting emotional language, and managing common challenges.
- Inter-Annotator Agreement (IAA): Measuring the level of agreement between different annotators on the same data points helps identify and address inconsistencies. Techniques like double annotation and kappa coefficient calculation can be used to ensure a minimum level of agreement.
- Leveraging Technology: While manual annotation remains crucial, leveraging technology can streamline the process. Annotation tools can offer features like pre-labeled templates, automatic sentiment scoring suggestions, and real-time feedback mechanisms to improve annotator accuracy and efficiency.
Conclusion:
Data annotation for sentiment analysis plays a pivotal role in unlocking the potential of this technology. By acknowledging the inherent challenges and implementing appropriate strategies, one can ensure the quality and comprehensiveness of annotated data, leading to more robust and accurate sentiment analysis models.
This, in turn, opens doors to diverse applications that can help businesses better understand their customers, improve product development, optimize marketing strategies, and ultimately foster deeper connections with stakeholders.