Artificial intelligence is slowly becoming a boon for the advancement of society. Take the presence of AI in the industrial sector for example. Industrial AI is revolutionizing how we manage and maintain equipment. Relying on the power of machine learning to analyze data and predict potential equipment failures before they occur, the industry can see wonderful developments. However, the success of these models hinges on a crucial but often overlooked step: data labeling.
Why is Data Labeling Important in Industrial AI?
Imagine an AI trying to learn a language without a dictionary. Sensor data from industrial equipment is a complex language filled with numbers, patterns, and subtle variations. Data labeling acts as the translator, providing labels and context that allows the AI to understand the data and identify the precursors to equipment failure.
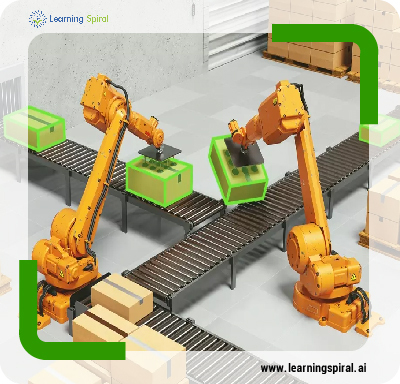
There are several ways data labeling is used in predictive maintenance:
- Image and Video Annotation: Industrial processes often involve visual inspections. Images and videos from cameras or drones can be annotated to identify specific components, defects like cracks or corrosion, and their severity. This helps the AI learn to recognize these patterns and predict when failures are likely.
- Sensor Data Annotation: Sensors embedded in equipment capture a wealth of data on vibration, temperature, pressure, and other parameters. Annotating these data points helps the AI identify normal operating ranges and detect anomalies that could signal impending problems.
- Time Series Annotation: Predictive maintenance often involves analyzing trends over time. Annotating sensor data with timestamps allows the AI to learn how equipment performance changes over its lifespan and identify deviations that indicate potential breakdowns.
Benefits of High-Quality Data Labeling
Investing in high-quality data labeling for your industrial AI project unlocks several benefits:
- Improved Model Accuracy: Clean and well-annotated data allows the AI to learn the nuances of equipment behavior. This translates to more accurate predictions, reducing the risk of false alarms and missed failures.
- Reduced Downtime: Accurate predictions enable proactive maintenance, allowing you to schedule repairs before equipment fails. This minimizes downtime and keeps your operations running smoothly.
- Lower Maintenance Costs: By focusing on targeted repairs instead of routine maintenance, you can optimize maintenance schedules and reduce overall costs.
- Extended Equipment Life: Predictive maintenance allows you to address issues early on, preventing further damage and extending the lifespan of your equipment.
Challenges of Data Labeling in Industrial AI
Data labeling for industrial AI presents its own set of challenges:
- Domain Expertise: Annotators need a deep understanding of the specific equipment and its failure modes. This may require collaboration between data scientists, engineers, and domain experts.
- Data Volume and Variety: Industrial processes can generate vast amounts of data in various formats, requiring a scalable annotation strategy.
- Data Privacy and Security: Industrial data often contains sensitive information. Secure data storage and annotation processes are crucial.
Strategies for Effective Data Labeling
Here are some key strategies for successful data labeling in industrial AI:
- Develop Clear Annotation Guidelines: Define the specific information you want annotators to capture to ensure consistency and accuracy.
- Utilize Specialized Tools: Data labeling platforms can streamline the process, manage large datasets, and provide quality control mechanisms.
- Leverage Active Learning: Start with a small, well-annotated dataset and iteratively refine it as the AI learns and identifies areas needing further annotation.
Conclusion
Data labeling plays a vital role in unlocking the full potential of predictive maintenance in industrial AI. By investing in high-quality annotated data, you can build accurate and reliable models that optimize your operations, reduce costs, and ensure the smooth running of your industrial processes.