As machine learning, aka ML applications, become advanced and spread worldwide, from facial recognition software to self-driving cars, ethical considerations in their development become more important. But, unlike common knowledge, the journey to ethics begins long before algorithms crunch data – it starts with the very foundation: data annotation.
Data annotation, the process of labeling and classifying data for ML training, often goes unseen, yet it holds immense power. The biases, inconsistencies, and inaccuracies embedded in annotated data can silently disturb the algorithms it feeds, leading to discriminatory or harmful outcomes.
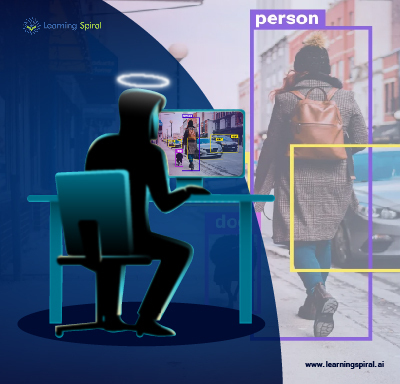
That’s why taking care of accurate data and providing sources for annotation is extremely important.
Here are some key ethical considerations in data annotation for ML applications:
1. Bias and Fairness:
Annotators, especially human ones, hold unconscious biases based on their background, experiences, and cultural context. These biases can easily seep into the labeling process, leading algorithms to discriminate against certain demographics or perpetuate existing societal inequalities.
To mitigate this, diverse annotation teams, rigorous bias detection measures, and continuous audits are crucial.
2. Privacy and Security:
Data often contains sensitive information like faces, voices, or personal attributes. Annotators must be trained on data privacy best practices and platforms should implement robust security measures to prevent data breaches and misuse.
Transparency regarding data usage and user consent are also essential ethical considerations.
3. Quality and Accuracy:
Inaccurate or inconsistent annotations can lead to unreliable and even dangerous ML models. Implementing quality control measures like double-checking labels, employing subject matter experts, and utilizing active learning techniques that prioritize informative data points are crucial.
4. Transparency and Explainability:
With AI decisions impacting critical areas like employment or healthcare, understanding how models arrive at their conclusions becomes paramount.
Transparent annotation processes and explainable AI techniques can offer an insight into the decision-making process, building trust and mitigating societal concerns about AI uncertainties.
5. Environmental Impact:
Large-scale data annotation often relies on energy-intensive infrastructure and hardware. Choosing energy-efficient solutions, promoting distributed annotation methodologies, and offsetting carbon emissions are crucial steps towards building sustainable and environmentally conscious AI.
Addressing these ethical challenges is not solely a technical endeavor; it demands a shift in mindset. Annotators need to be treated as skilled professionals, not just cheap labor. Fair compensation, ethical training, and ongoing support are essential to ensure the integrity and well-being of the human workforce behind the data curtain.
The responsibility of ethical data annotation doesn’t fall solely on annotators or developers; it extends to researchers, policymakers, and consumers. Building ethical frameworks, regulating data practices, and demanding transparency from technology companies are all steps towards a future where AI serves as a force for good, not a mirror reflecting our biases and inequalities.
By taking a conscious and proactive approach to data annotation, we can ensure that the foundations of AI are built on principles of fairness, transparency, and responsibility, paving the way for a future where everyone benefits from the power of intelligent machines.