Data annotation, the process of labeling raw data for machine learning algorithms, is a critical component of artificial intelligence development. While it plays a vital role in training models to perform various tasks, ethical considerations must be carefully addressed to ensure responsible and fair AI.
In this article, we will discuss the different parameters of this area.
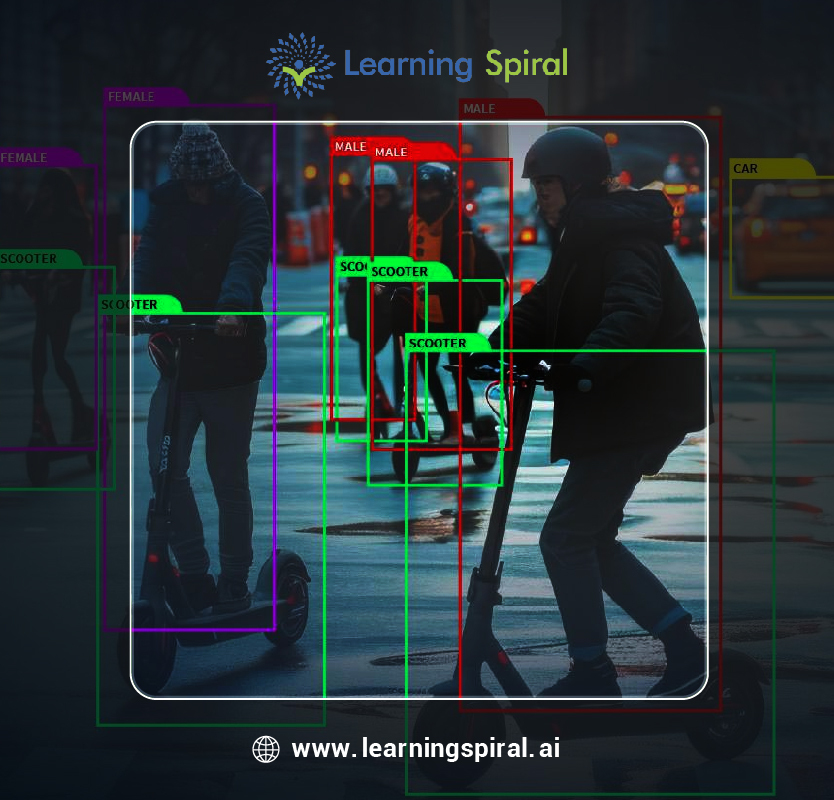
- Bias and Discrimination
One of the most pressing ethical concerns in data annotation is the potential for bias to be introduced into the dataset. If the training data is not representative of the real world or contains biases, the resulting model may perpetuate or amplify existing discrimination.
For example, a facial recognition system trained on a dataset primarily consisting of images of white individuals may struggle to accurately identify people of color.
To mitigate bias, it is essential to ensure that the data annotation process is inclusive and diverse. This involves collecting data from a wide range of individuals, considering factors such as race, gender, age, and socioeconomic status. Additionally, annotators should be trained to recognize and avoid biases in their labeling.
- Privacy and Data Protection
Protecting the privacy of individuals whose data is used for annotation is another crucial ethical consideration. Sensitive information, such as personal identifiers, medical records, or financial data, must be handled with care.
Data anonymization or pseudonymization techniques can be employed to reduce the risk of privacy breaches.
Furthermore, it is important to obtain informed consent from individuals before using their data for annotation. This involves clearly explaining the purpose of the project, how their data will be used, and the potential risks and benefits.
- Quality and Accuracy
The quality and accuracy of annotated data directly impact the performance of the resulting AI model. Inaccurate or inconsistent labeling can lead to biased or unreliable outcomes. Therefore, it is essential to implement quality control measures to ensure the accuracy of annotations.
This may involve using multiple annotators to verify labels, employing automated tools to detect inconsistencies, and providing clear guidelines and training to annotators.
- Transparency and Accountability
Transparency and accountability are essential for building trust in AI systems. Organizations involved in data annotation should be transparent about their data collection and labeling practices.
This includes disclosing the sources of data, the methods used for annotation, and any potential biases.
Additionally, there should be mechanisms in place for accountability, ensuring that individuals and organizations are held responsible for any negative consequences arising from the use of biased or inaccurate data.
- Fairness and Equity
AI systems should be developed and deployed in a way that is fair and equitable. This means that the benefits and risks of these systems should be distributed equitably across different populations.
Data annotation plays a crucial role in ensuring fairness by ensuring that the training data is representative of the diverse population that the model will serve.
Conclusion
Ethical considerations in data annotation are essential to ensure the responsible and fair development of AI. By addressing issues such as bias, privacy, quality, transparency, and fairness, organizations can help to build AI systems that benefit society and avoid harmful consequences.