Aerial imagery, captured from drones, satellites, or airplanes, has become an indispensable tool for various industries. To unlock the full potential of this data, image annotation plays a crucial role. This process involves labeling objects, features, or areas within aerial images to provide context and structure.
Key Applications of Aerial Image Annotation
Here are the following places where image annotation works wonder in aerial applications:
- Urban Planning: Identifying and mapping infrastructure, buildings, and land use patterns for urban development and planning.
- Environmental Monitoring: Tracking deforestation, pollution, and natural disasters.
- Agriculture: Assessing crop health, monitoring irrigation, and detecting anomalies in agricultural fields.
- Infrastructure Inspection: Inspecting power lines, roads, and bridges for damage or maintenance needs.
- Autonomous Vehicles: Training AI models for autonomous vehicles to navigate complex urban environments.
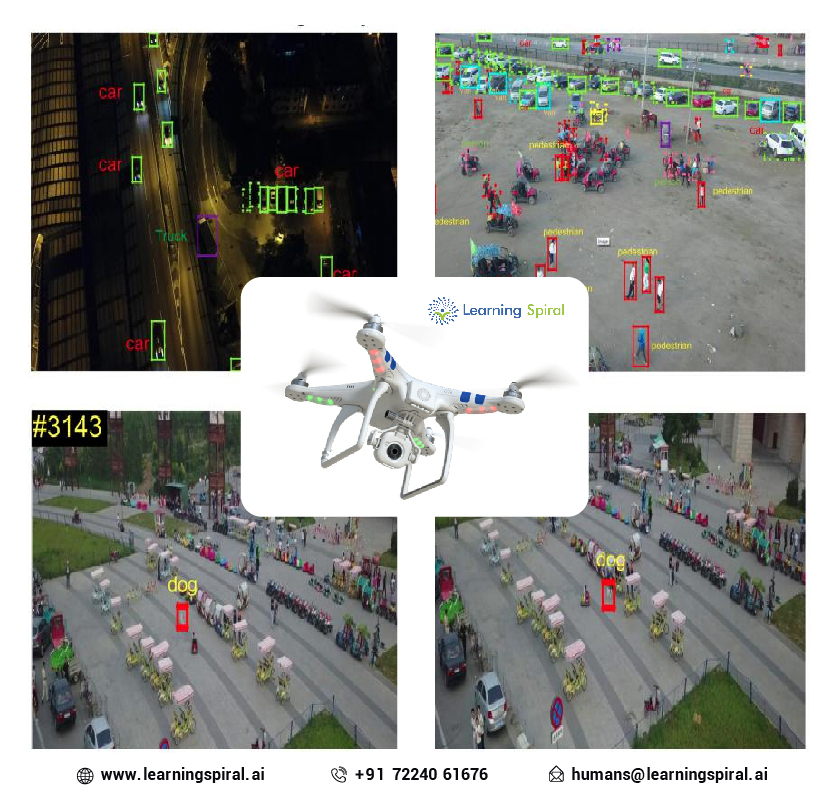
Types of Aerial Image Annotation
Following are the various types of image annotation under aerial segment:
- Object Detection: Identifying and labeling specific objects within the image, such as vehicles, buildings, or trees.
- Semantic Segmentation: Assigning labels to every pixel in the image, categorizing them into different classes like road, sidewalk, or vegetation.
- Instance Segmentation: Differentiating between individual instances of the same object, such as multiple cars or buildings.
- Attribute Recognition: Identifying specific attributes of objects, like the color of a building or the type of vegetation.
Challenges and Considerations
- Image Quality:
Aerial images can be affected by factors like weather conditions, altitude, and sensor quality. These factors can impact the accuracy of annotation.
- Data Volume:
The vast amount of aerial data generated can make manual annotation time-consuming and costly.
- Annotation Complexity:
Complex scenes with overlapping objects or subtle details can pose challenges for annotators.
- Data Privacy:
Ensuring compliance with data privacy regulations when annotating aerial images, especially those containing sensitive information.
Advancements in Aerial Image Annotation
- Automated Annotation Tools: The development of AI-powered tools can automate parts of the annotation process, improving efficiency and accuracy.
- Active Learning: Techniques like active learning can prioritize the most informative images for annotation, reducing the overall workload.
- Transfer Learning: Leveraging pre-trained models on large datasets can accelerate the training of new models for specific aerial image annotation tasks.
- Crowdsourcing: Utilizing platforms like Amazon Mechanical Turk to recruit a large number of annotators can help address data volume challenges.
Future Trends
- 3D Annotation: As drone technology advances, 3D annotation will become increasingly important for tasks like urban planning and infrastructure inspection.
- Real-time Annotation: Real-time annotation of aerial images will enable immediate analysis and decision-making in applications like disaster response.
- Integration with Other Data Sources: Combining aerial imagery with other data sources, such as satellite data or ground-based sensors, can provide a more comprehensive understanding of the environment.
In conclusion, aerial image annotation is a critical component of many advanced applications. By addressing the challenges and leveraging emerging technologies, we can unlock the full potential of aerial data to drive innovation and improve decision-making in various fields.