Natural Language Processing (NLP) is revolutionizing industries by enabling machines to understand and process human language. However, the effectiveness of NLP models depends on high-quality annotated text data. Data annotation for NLP plays a crucial role in training AI algorithms, ensuring accuracy in tasks like machine translation, sentiment analysis, and chatbots. This article explores key NLP data annotation strategies and best practices that enhance AI-powered language models.
Understanding NLP Data Annotation 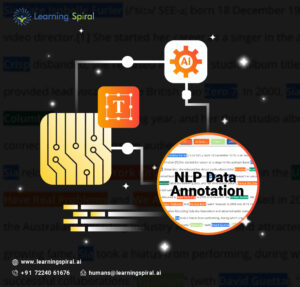
NLP data annotation is the process of labeling text data to train machine learning models. This involves tasks like entity recognition, sentiment labeling, part-of-speech tagging, and intent classification. Without proper annotation, NLP models struggle to achieve accuracy, making data labeling services vital for AI development.
Key Strategies for Annotating NLP Data
To maintain consistency, it is essential to establish data annotation guidelines outlining labeling rules, examples, and edge cases. This reduces ambiguity and ensures that annotators correctly interpret the text data. Leveraging AI-driven pre-annotation tools speeds up the annotation process. These tools use pre-trained NLP models to suggest labels, allowing human annotators to verify and correct errors. This hybrid approach improves efficiency while maintaining high accuracy.
Different annotation techniques serve different NLP tasks. Named Entity Recognition (NER) identifies entities like names, locations, and organizations, while sentiment annotation labels text as positive, negative, or neutral. Intent classification categorizes user queries for virtual assistants, and part-of-speech tagging assigns grammatical categories to words. Combining these techniques enhances training datasets and strengthens machine learning annotation models.
Even with automated tools, human intervention is essential. Implementing a quality assurance process where multiple annotators review the same dataset minimizes bias and improves accuracy. To manage large datasets, using scalable data annotation services like Learning Spiral AI is crucial. Our AI-powered text annotation solutions provide scalable, high-precision NLP training data for businesses worldwide.
Bias in annotation can skew NLP models. Using diverse annotator teams and cross-verifying data helps mitigate this risk, ensuring a balanced dataset that represents various linguistic and cultural contexts. Outsourcing text data annotation to experts like Learning Spiral AI ensures high-quality labeled datasets at reduced costs. Our skilled annotators deliver reliable data labeling for NLP, helping businesses build superior AI models.
Conclusion
NLP data annotation is a critical component of AI development, requiring precise labeling strategies to train effective language models. By adopting best practices like clear guidelines, pre-annotation tools, and human review, businesses can improve the quality of their NLP datasets. At Learning Spiral AI, we provide AI-powered annotation services tailored for machine learning NLP projects. Partner with us to access high-quality NLP training data and accelerate your AI innovations. Contact us today to scale your NLP data annotation needs!