As Artificial Intelligence (AI) continues its exponential growth, the importance of data annotation takes center stage. This meticulous process of tagging and labeling images is the lifeblood of AI development, feeding data to machine learning models and enabling them to “learn.”
However, the ethical considerations surrounding image annotation require careful attention, particularly concerning data privacy and worker rights.
Data Privacy Concerns:
The images used for annotation often contain sensitive information. Personal details like faces, license plates, and even home exteriors can be present in everyday scenes. When these images are used for AI development, it is crucial to ensure the privacy of the individuals depicted.
- Data Anonymization: Techniques like blurring faces, redacting license plates, and anonymizing locations can go a long way in protecting individual privacy.
- Informed Consent: In cases where identifiable information is unavoidable, obtaining explicit consent from individuals depicted in the images is paramount.
- Data Security: Robust data security measures must be implemented to prevent unauthorized access or misuse of sensitive image data. Regulations like the GDPR (General Data Protection Regulation) in Europe and CCPA (California Consumer Privacy Act) in the US provide frameworks for data privacy compliance.
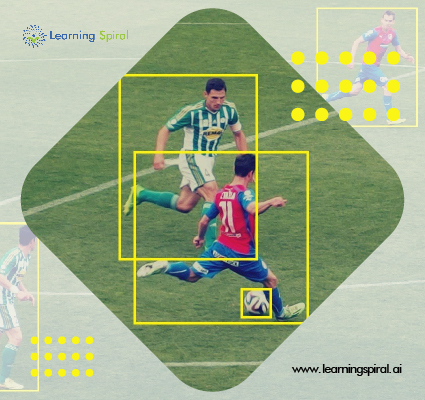
Ensuring Worker Rights:
Data labelers, the individuals who perform the critical task of image annotation, deserve fair treatment and ethical working conditions.
- Fair Compensation: Data labelers should be compensated fairly for their time and expertise. The work can be intricate and requires focus, and compensation models should reflect this.
- Training and Support: Data labelers require proper training on annotation guidelines, data privacy protocols, and ethical considerations. Additionally, they should have access to ongoing support and resources.
- Transparency and Communication: Clear communication channels between data labeling companies and workers are essential. Data labelers have the right to understand how their work contributes to the final AI product and how their data is used.
- Respectful Work Environment: Data labeling companies should foster a respectful and inclusive work environment for all participants in the annotation process.
Mitigating Bias:
The data used for training AI models can inadvertently perpetuate societal biases. Image annotation tasks are not immune to this risk. Bias can creep in through:
- Data Selection: Ensuring diverse datasets that represent various demographics and scenarios helps mitigate bias.
- Worker Backgrounds: Having a diverse pool of data labelers from different backgrounds can help reduce the influence of individual biases.
- Standardized Annotation Guidelines: Clear and consistent annotation guidelines minimize the risk of subjective interpretations that might introduce bias.
Transparency and Accountability:
There must be transparency in how image annotation is conducted and how the resulting data is used. This promotes accountability and fosters trust with the public.
- Data Usage Disclosure: Companies should be transparent about the intended use of annotated image data and how it contributes to the final AI product.
- Responsible AI Development: The core principles of responsible AI development, such as fairness, accountability, transparency, and human oversight, should be integrated into the image annotation process.
Conclusion:
The future of AI is inextricably linked to ethical image annotation practices. By prioritizing data privacy, ensuring worker rights, and mitigating bias, we can cultivate a responsible AI ecosystem that benefits society as a whole.
Addressing these ethical considerations is not just a moral imperative but also a necessity for building trust in AI and ensuring it serves humanity for the greater good.