With the development of technology, the biggest threat to a person’s personal life was the advancing nature of the tech, making keeping things private difficult. To avoid this phenomenon, a new kind of security was released, which we know today as facial recognition. Facial recognition technology, with its promises of enhanced security and convenience, is rapidly weaving its way into our lives.
But behind the scenes lies a crucial process – data annotation, the task of meticulously labeling and classifying facial images to train recognition algorithms. While this process fuels the technology’s progress, it also raises critical questions about privacy and security.
In this article, we will discuss the considerations needed for privacy and security areas while annotating data for facial recognition.
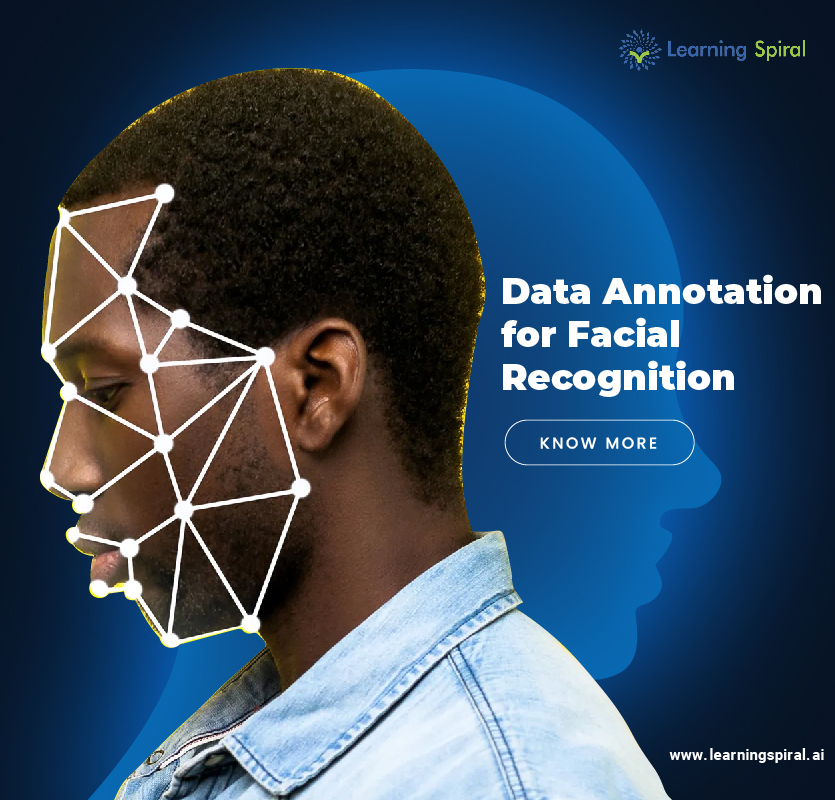
Privacy Considerations:
Data annotation for facial recognition often involves collecting and processing vast amounts of personal data, including high-resolution images and demographic information. This raises immediate concerns about individual privacy. Without proper safeguards, these images could be misused for purposes beyond their intended scope, leading to potential stalking, discrimination, and even profiling.
Further, the very nature of facial data makes it uniquely sensitive. Unlike passwords or credit card numbers, faces cannot be easily changed or replaced. A data breach involving facial information could have lasting consequences, jeopardizing an individual’s security and well-being.
To avoid this, elements such as pseudonymization, de-identification, and differential privacy techniques are utilized.
Security Considerations:
The data annotation process itself presents security vulnerabilities. The centralized storage of vast image libraries creates tempting targets for hackers. A successful breach could expose a trove of sensitive data, putting countless individuals at risk.
Additionally, the human element in annotation introduces potential vulnerabilities. Malicious actors, posing as annotators, could inject misleading or biased data into the system, compromising the accuracy and integrity of the facial recognition model.
For this encryption, access controls and secure platforms are implemented.
Navigating this privacy and security labyrinth requires a multi-pronged approach. Here is a brief insight into it:
- Transparency and Consent: Building trust is crucial. Organizations involved in data annotation must be transparent about their practices, clearly informing individuals about how their data will be collected, used, and stored.
- Data Minimization and Anonymization: Collecting and storing only the minimum amount of data necessary for training purposes is essential. Additionally, anonymizing or de-identifying data wherever possible can further mitigate privacy risks.
- Robust Security Measures: Implementing robust security measures such as encryption, access controls, and intrusion detection systems is critical to protect data from unauthorized access. Moreover, regularly conducting security audits can identify and address potential vulnerabilities.
- Independent Oversight and Accountability: Establishing independent oversight mechanisms can ensure compliance with data privacy regulations and ethical guidelines. Holding all actors accountable for responsible data handling is crucial.
Conclusion
The promise of facial recognition technology is undeniable. However, its development and deployment must proceed cautiously toward privacy and security. By prioritizing transparency, minimizing data collection, and implementing robust safeguards, we can ensure that data annotation fuels this powerful technology’s responsible advancement without compromising individuals’ fundamental rights and well-being.