Data annotation is a critical step for artificial intelligence’s development as it involves labeling and tagging data to train algorithms. Among its types, crowdsourcing data annotation is an essential variety, which involves outsourcing the labeling task to a large group of contributors instead of hiring fixed annotators.
Crowdsourcing data annotation has gained popularity as it is cost-effective and easily scalable. However, it comes with its own set of advantages and challenges. Let’s explore the different areas along with the best practices associated with crowdsourcing data annotation.
Pros & Cons of Crowdsourcing Data Annotation:
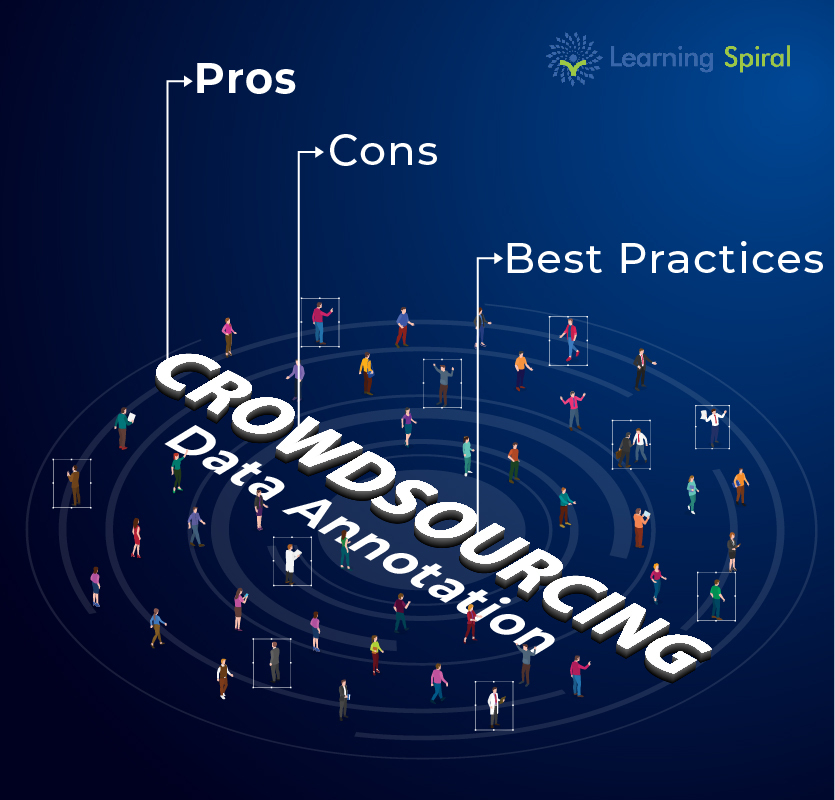
Here is a list of the advantages that make crowdsourcing so appealing:
- Cost-Effectiveness: One of the primary advantages of crowdsourcing is cost savings. By tapping into a global pool of annotators, you can often label data at a fraction of the cost of hiring in-house annotators or a specialized team.
- Scalability: Crowdsourcing allows you to scale up annotation efforts quickly. You can engage a large workforce to handle substantial datasets within tight deadlines.
- Speed: With a large workforce, you can accelerate the annotation process, helping your project move forward at a rapid pace.
- Flexibility: Crowdsourcing platforms often allow for flexibility in terms of task allocation, enabling you to adapt to changing project needs and priorities.
Cons of Crowdsourcing Data Annotation:
Here is the list of disadvantages that constrict crowdsourcing from being the best annotation option:
- Quality Control: Maintaining annotation quality can be challenging when dealing with a diverse group of annotators. Ensuring accuracy, consistency, and adherence to guidelines is crucial.
- Data Privacy and Security: Sharing data with external annotators may pose privacy and security risks. Careful management and anonymization of sensitive data are essential.
- Communication Challenges: Effective communication and clarification of guidelines can be more complex when working with remote, often anonymous, annotators. Misinterpretations or questions may arise.
- Worker Turnover: Crowdsourcing platforms often have high turnover rates, which can lead to inconsistencies and additional training efforts as new annotators are onboarded.
Best Practices for Crowdsourcing Data Annotation:
Here’s how to practice crowdsourcing in a more efficient manner:
- Clear and Detailed Guidelines: Provide comprehensive annotation guidelines that are easy to understand.
- Quality Control: Implement a robust quality assurance process involving random checks and continuous feedback loops.
- Regular Training: Continuously train annotators to improve their understanding of the task, data, and annotation guidelines.
- Data Security: Prioritize data security by anonymizing sensitive information and selecting trustworthy crowdsourcing platforms that adhere to data privacy regulations.
- Effective Communication: Maintain open lines of communication with annotators to address questions and concerns. Encourage feedback and provide a means for annotators to seek clarification.
- Task Segmentation: Divide large datasets into smaller, manageable tasks to minimize the risk of errors due to fatigue and maintain annotator engagement.
- Pilot Testing: Begin with a pilot project to identify potential issues and refine guidelines before launching large-scale annotation efforts.
- Incentives: Offer fair compensation and incentives to retain experienced annotators and reduce turnover.
- Continuous Evaluation: Regularly evaluate the performance of annotators and adjust your quality control process as needed.
- Monitoring and Feedback: Monitor the annotation process in real time and provide immediate feedback to annotators when errors are detected.
Crowdsourcing data annotation can be a powerful tool for handling large datasets efficiently and cost-effectively. By following the above-mentioned data practices, one can reduce the cons of crowdsourcing and monetize on the pros.