Image annotation, the process of labeling objects or features within an image, is becoming increasingly crucial in the retail industry. As retailers leverage artificial intelligence (AI) to enhance customer experiences and optimize operations, accurate and well-annotated images play a pivotal role.
This article explores the significance of image annotation in retail, highlighting its applications and challenges.
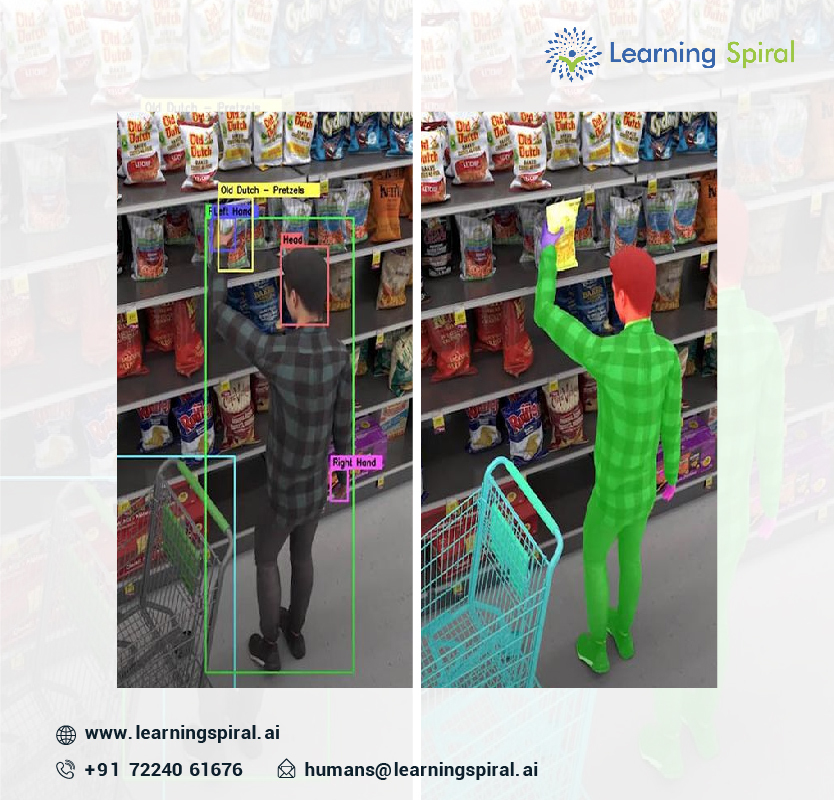
Applications of Image Annotation in Retail
Image annotation has a wide range of applications in the retail sector:
- Product Categorization: Automating the process of categorizing products based on their visual attributes.
- Visual Search: Enabling customers to search for products using images as queries.
- Inventory Management: Accurately tracking product stock levels and identifying discrepancies.
- Personalized Recommendations: Suggesting products to customers based on their preferences and browsing history.
- Quality Control: Detecting defects or inconsistencies in products during production or shipping.
Key Challenges in Image Annotation
While image annotation offers numerous benefits, it also presents several challenges:
- Data Quality: Ensuring high-quality images with clear and consistent labeling is essential for accurate AI models.
- Annotation Complexity: Complex images with multiple objects or occlusions can be challenging to annotate.
- Labeling Consistency: Maintaining consistency among annotators is crucial for accurate results.
- Scalability: Handling large volumes of images efficiently can be a bottleneck.
- Privacy Concerns: Protecting customer privacy when annotating images that contain personally identifiable information.
Best Practices for Image Annotation in Retail
To overcome these challenges and maximize the benefits of image annotation, retailers should follow these best practices:
- Clear Guidelines: Provide clear and concise guidelines to annotators, including definitions of object categories and labeling conventions.
- Quality Control: Implement quality control measures to ensure accuracy and consistency in annotations.
- Data Augmentation: Use techniques like rotation, flipping, and cropping to increase the diversity of the training dataset.
- Annotation Tools: Leverage advanced annotation tools that streamline the process and improve efficiency.
- Privacy Considerations: Implement measures to protect customer privacy, such as anonymizing images or obtaining explicit consent.
Emerging Trends in Image Annotation
Several emerging trends are shaping the future of image annotation in retail:
- Active Learning: Using AI to select the most informative images for annotation, reducing the overall workload.
- Federated Learning: Training AI models on decentralized data, ensuring privacy while improving accuracy.
- Synthetic Data: Generating synthetic images to augment training datasets and address data scarcity.
- Automated Annotation: Developing automated tools to reduce the manual effort required for annotation.
Conclusion
Image annotation is a critical component of AI-powered retail solutions. By overcoming challenges and adopting best practices, retailers can leverage image annotation to improve customer experiences, optimize operations, and gain a competitive edge.
As technology continues to advance, we can expect to see even more innovative applications of image annotation in the retail industry.