Artificial intelligence (AI) increasingly relies on visual information from self-driving cars to facial recognition software. Just like humans need labels to understand the world around them, AI models also require video annotation to grasp the nuances of video content.
Hence, video annotation is considered one of the most important data annotations among its peers. Let’s delve into video annotation, uncovering its crucial role in training machine learning (ML) models.
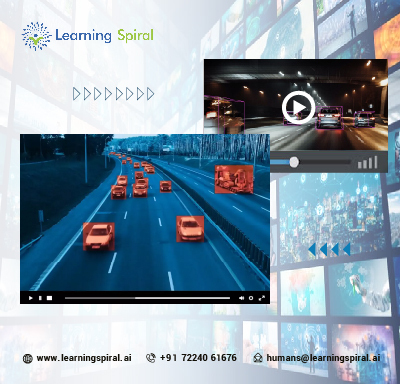
What is Video Annotation?
Video annotation is the process of adding labels and metadata to video frames. These labels can categorize objects (cars, people, animals), track their movement, describe actions (walking, running, interacting), or even capture emotions. Essentially, you’re providing the AI model with a “cheat sheet” to interpret the visual world.
Types of Video Annotation:
Following are the major types of video annotation utilized in the market:
- Object Detection & Classification: Annotators identify and label specific objects present in each frame.
- Object Tracking: The movement and location of objects are tracked across consecutive frames.
- Scene Description: Tags capture the overall context of the scene (e.g., street, park, office).
- Action Recognition: Specific actions performed by objects are identified and labeled.
- Sentiment Analysis: Emotional states portrayed in the video are tagged.
Why is Video Annotation Crucial for AI?
Imagine training an AI model to identify different dog breeds without any labels. The model would be lost in a sea of furry pixels. Video annotation provides the structure and context AI needs to learn:
- Improved Accuracy: Labeled data helps AI models distinguish similar objects or actions, leading to higher accuracy in tasks like object detection and activity recognition.
- Real-World Applicability: Annotations cater to specific scenarios, ensuring the AI model functions effectively in nuanced real-world situations.
- Reduced Bias: Diversely annotated data minimizes the risk of biased algorithms that discriminate against certain groups or objects.
- Faster Development: Labeled datasets accelerate the development process by providing readily available training data for AI models.
The Challenges of Video Annotation:
Despite its importance, video annotation presents several challenges:
- Cost & Time: Annotating vast amounts of video data can be expensive and time-consuming, especially for complex tasks.
- Data Quality: Inconsistent or inaccurate annotations can lead to flawed AI models.
- Privacy Concerns: Data privacy regulations add complexity to the annotation process, especially when dealing with personally identifiable information.
Emerging Solutions:
Thankfully, technology is evolving to address these challenges:
- Automated Annotation Tools: AI-powered tools can automate some labeling tasks, speeding up the process and reducing costs.
- Crowd-Sourcing Platforms: Distributed networks of annotators can handle large datasets efficiently.
- Privacy-Preserving Techniques: Secure labeling techniques safeguard sensitive information while enabling effective annotation.
Conclusion:
As AI applications expand, video annotation will continue to play a vital role. Advancements in automation, crowdsourcing, and privacy-preserving techniques will make this process more efficient, cost-effective, and secure.
With continued innovation, video annotation can unlock the full potential of AI, paving the way for a future where machines truly “see” the world around them.